What Synthetic Data Means for the Future of Waste Sorting


Every second counts in the bustling environment of a waste sorting facility, where conveyors hum and materials blur past at high speed. Decisions—hundreds, maybe thousands of them—are made in the blink of an eye. Is that piece of plastic recyclable? Is this metal fragment worth recovering? At Waste Robotics, we're constantly asking ourselves: how can we make these decisions faster, smarter, and more precise? The answer lies in AI models—but not just any models. It's a journey that starts with the giants of the AI world: foundation models.
The Backbone of Intelligence
Foundation models are the heavyweight champions of AI. Trained on colossal datasets, these models know little about many things. They're versatile and capable of understanding images, text, and sometimes even speech. Think of them as the encyclopedias of artificial intelligence—packed with knowledge but requiring some personalization to fit specific needs. At Waste Robotics, we harness these foundation models to train smaller, leaner, and faster systems designed specifically for waste sorting. It's like starting with a broad base of knowledge and refining it into laser-focused expertise. But why not just use these massive models directly?
A Balancing Act: The Pros and Cons
Foundation models are undeniably powerful, but they come with baggage. Training and running these models demands immense computational resources—not ideal when you need quick decisions on a busy conveyor belt. They're also prone to carrying biases from their training data, which can sometimes lead to suboptimal outcomes. On the flip side, their strengths are hard to ignore. They provide a starting point, a scaffolding upon which we can build something faster and more tailored. That's where Waste Robotics steps in, with a unique approach that blends innovation with efficiency.
The Role of Synthetic Images
Here's where the story gets exciting. To train our AI, we need images—lots of them. However, capturing every possible variation of waste materials in real-life settings is neither practical nor cost-effective. Enter synthetic images. Using advanced generative models, we create high-quality, computer-generated images of waste materials. These aren't just placeholders; they're diverse, realistic, and tailored to simulate the complexities of real-world waste. From crushed cans to folded cardboard, we ensure our AI sees it all before it encounters the real thing. This synthetic data not only speeds up the training process but also makes it more robust. We can control every variable—lighting, angle, material type—to ensure our models are ready for anything.
Why Smaller and Faster Models Matter
The result? Smaller, faster AI models that thrive in the chaos of a waste sorting facility. These models are agile enough to process decisions in real-time, ensuring materials are sorted with precision. They're also less resource-intensive, making them cost-effective and more accessible to deploy across various facilities. Speed and accuracy aren't just nice-to-haves in waste-sorting robotics—they're non-negotiables. By scaling down the size of our models without compromising their intelligence, we create systems that work smarter, not harder.
Looking Ahead
The future of waste sorting robotics is exciting. As synthetic image generation technology evolves, the possibilities are endless. Imagine training AI to recognize entirely new materials or adapt to regional variations in waste composition—all before these changes even occur in the real world. Emerging trends, such as multimodal AI and more sophisticated generative models, promise to make our systems more adaptive and efficient. At Waste Robotics, we're not just following these advancements—we're helping to shape them.
Closing the Loop
In the end, our work is about more than just technology. It's about creating systems that help the waste management industry run more efficiently and sustainably. By blending the power of foundation models with the ingenuity of synthetic data and the precision of smaller, faster AI systems, we're redefining what's possible in waste sorting robotics. The conveyor belt keeps moving, and so do we—always looking for the next innovation to help turn waste into opportunity.
Subscribe to Our Newsletter
Related posts
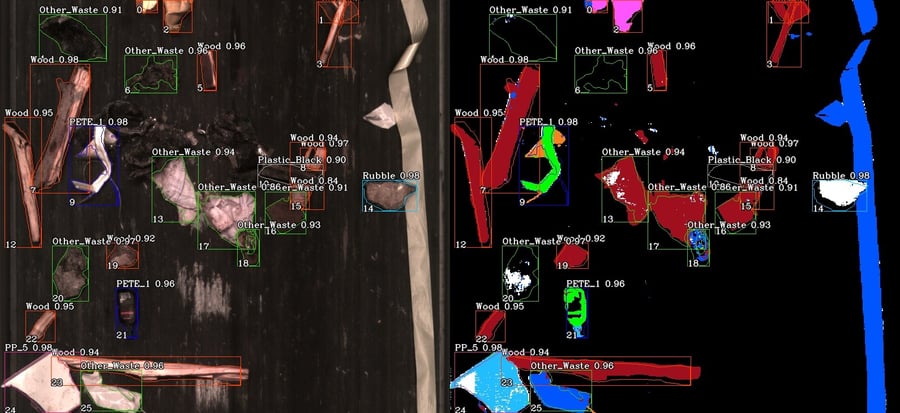
Breaking Barriers in Waste Sorting: How AI is Tackling Object Detection Challenges
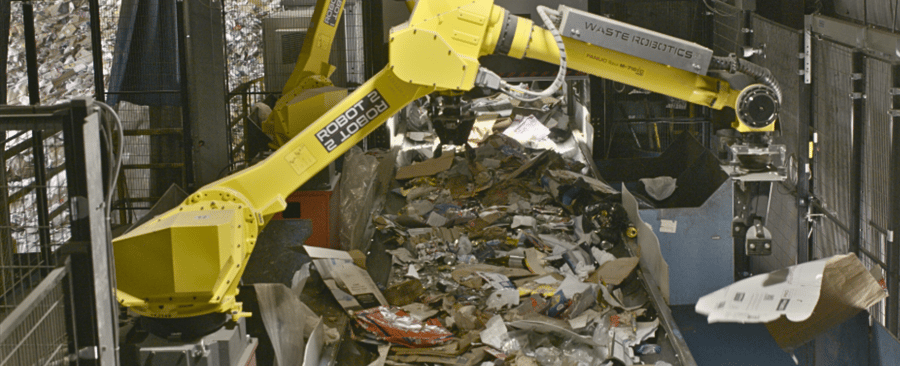